In today's interconnected financial landscape, Anti-Money Laundering (AML) practices play a critical role in maintaining the integrity of the global financial system. AML efforts are essential for detecting and preventing illicit activities such as money laundering, terrorist financing, and other financial crimes that can undermine economic stability and security.
However, the landscape of money laundering is continuously evolving, presenting new challenges and complexities for financial institutions and regulatory bodies. Traditional AML tactics, while foundational, are increasingly being tested by sophisticated laundering schemes, the rise of digital currencies, and the globalization of financial transactions. As criminals adopt more advanced methods, the need for innovative and forward-thinking AML strategies becomes paramount.
The Limitations of Traditional AML Tactics
For decades, financial institutions have relied on traditional AML methods to detect and prevent money laundering activities. While these traditional AML tactics have been foundational in the fight against money laundering, they come with several significant challenges and limitations:
- High False Positive Rates: Rule-based systems often flag legitimate transactions as suspicious, overwhelming compliance teams and diverting resources from genuine threats.
- Manual Review Bottlenecks: Reliance on manual reviews slows down investigations, as human analysts can only process a limited number of alerts, leading to delays and potential oversight.
- Inflexibility and Scalability Issues: Static rule-based systems struggle to adapt to evolving money laundering techniques and are costly to scale for large transaction volumes.
- Limited Data Integration: Operating in silos, traditional methods often fail to integrate data from various sources, resulting in incomplete risk assessments and missed connections.
- Reactive Rather Than Proactive: Traditional tactics identify suspicious activities after they occur, limiting real-time prevention and allowing illicit funds to move through the system.
- Regulatory Pressure and Compliance Costs: Increasing regulatory demands require significant investment in technology, personnel, and training, adding to the overall cost of AML programs.
- Complexity of Global Transactions: The globalization of financial transactions complicates tracking and analysis, especially across multiple jurisdictions and regulatory frameworks.
Given these limitations, it is clear that traditional AML tactics are insufficient for combating modern money laundering. The need for advanced technologies and innovative approaches is critical, as we will explore in the following sections.
Advanced AI and Machine Learning Models in AML
Artificial Intelligence (AI) and Machine Learning (ML) have significantly transformed AML practices. Initially used to enhance rule-based systems, these technologies now offer sophisticated, proactive solutions. AI and ML models can process vast amounts of data quickly, identifying patterns and anomalies that human analysts might miss. Over time, these models continuously learn and adapt, improving their accuracy and effectiveness.
Current State of AI and ML in AML
Today, AI and ML are integral to modern AML strategies, particularly among larger financial institutions and fintech companies. However, smaller institutions often lag in adoption due to budget constraints and a lack of technical expertise. According to a survey by the ACAMS, over 70% of AML professionals believe AI and ML will be crucial in the future of AML. Despite this optimism, challenges such as data quality, the need for specialized skills, and potential algorithmic bias remain.
AI/ML Models Used for Anomaly Detection and Pattern Recognition
- Supervised Learning Models: Trained on labeled datasets to classify transactions as legitimate or suspicious. Common algorithms include decision trees and logistic regression.
- Unsupervised Learning Models: Identify hidden patterns and anomalies in large datasets without labeled data. Clustering algorithms like K-means are often used.
- Semi-supervised Learning Models: Combine supervised and unsupervised learning, which are useful when labeled data is scarce.
- Neural Networks and Deep Learning: Effective for complex pattern recognition tasks. Models like Convolutional Neural Networks (CNNs) analyze sequential transaction data.
- Natural Language Processing (NLP): Analyzes unstructured data, such as transaction descriptions, to enhance AML accuracy.
- Graph-Based Models: Represent transactions and entities as nodes and edges in a graph, effective for detecting complex money laundering schemes.
- Anomaly Detection Algorithms: Algorithms like Isolation Forests identify transactions that deviate significantly from normal behavior.
Experts predict wider adoption as technology becomes more accessible and regulatory bodies endorse these methods. However, challenges such as data quality, the need for specialized skills, and algorithmic bias must be addressed. Financial institutions will need to invest in training and development to build the necessary expertise and ensure models are transparent and free from bias.
Blockchain and DLT in AML
Blockchain and Distributed Ledger Technology (DLT) are poised to revolutionize AML practices by offering unparalleled transparency, security, and traceability. These technologies provide a decentralized and immutable ledger, ensuring that transaction records are tamper-proof and reliable. Blockchain's transparent nature allows all network participants to view transaction histories, making it easier to track funds and identify suspicious activities. Additionally, the immutability of blockchain records guarantees data integrity, making them a dependable source for audits and investigations.
Key benefits of blockchain and DLT in AML include:
- Enhanced Transparency: Real-time visibility of transaction histories for all network participants.
- Immutable Records: Tamper-proof transaction data that ensures integrity and reliability.
- Smart Contracts: Automated compliance checks and real-time flagging of suspicious transactions.
- Cross-Border Transactions: Faster, more secure international transactions, simplifying the detection and prevention of cross-border money laundering schemes.
Experts are optimistic about the potential of blockchain and DLT in AML, although widespread adoption is still in its early stages due to regulatory and technical challenges. As these technologies mature and regulatory frameworks evolve, their role in AML is expected to grow, making them critical components of future AML strategies.
The Impact of Regtech on AML Compliance
Regulatory Technology, or Regtech, is transforming the landscape of AML compliance by leveraging advanced technologies to streamline and enhance regulatory processes. Regtech solutions are designed to help financial institutions comply with regulations more efficiently and effectively, reducing the burden of manual compliance tasks and minimizing the risk of non-compliance.
Regtech's significance in AML lies in its ability to automate and optimize various compliance functions, including transaction monitoring, risk assessment, and reporting. By utilizing technologies such as AI, ML, and big data analytics, Regtech tools can analyze vast amounts of data in real-time, identify suspicious activities, and ensure that institutions remain compliant with evolving regulatory requirements.
Specific Regtech tools and platforms for AML include:
- Automated KYC Solutions: These tools streamline the Know Your Customer (KYC) process by automating identity verification and risk assessment, reducing the time and effort required for manual checks.
- Transaction Monitoring Systems: Advanced transaction monitoring platforms use AI and ML to detect unusual patterns and flag suspicious transactions, improving the accuracy and efficiency of AML efforts.
- Regulatory Reporting Tools: These solutions automate the generation and submission of regulatory reports, ensuring timely and accurate compliance with reporting requirements.
- Risk Assessment Platforms: Regtech tools can assess the risk profiles of customers and transactions in real-time, enabling institutions to take proactive measures to mitigate potential risks.
In summary, Regtech is playing a crucial role in enhancing AML compliance by automating and optimizing various regulatory processes. As these technologies continue to evolve, their impact on AML efficiency and accuracy is expected to grow, making them indispensable tools for financial institutions striving to stay ahead in the fight against money laundering.
Future Trends in Regtech for AML
As we look to the future, several emerging trends are expected to shape the Regtech landscape for AML compliance:
- Regtech as a Service (RaaS): Cloud-based solutions will offer flexible, scalable compliance tools, reducing the need for significant upfront investments.
- Enhanced Data Analytics: Leveraging big data and advanced analytics will provide deeper insights into customer behaviors and transaction patterns, enabling more proactive AML measures.
- Interoperability and Integration: Future Regtech platforms will focus on seamless integration, creating unified ecosystems where different tools can work together efficiently.
- User-Centric Design: Next-gen Regtech solutions will prioritize intuitive, user-friendly interfaces to improve efficiency and reduce the learning curve for compliance teams.
- Regulatory Sandboxes: Controlled environments will allow for the testing of innovative compliance tools without regulatory penalties, fostering innovation.
The future of Regtech in AML compliance is set to be transformative. Integrating advanced solutions will be essential for staying ahead of regulatory changes and enhancing AML effectiveness.
Biometric Authentication and AML
Biometric authentication significantly reduces the risk of identity fraud by ensuring that only legitimate users can access financial services. By integrating biometrics into AML processes, financial institutions can more accurately verify customer identities, making it harder for criminals to use stolen or fake identities to launder money.
The future of biometric authentication in AML is promising, with several key trends emerging:
- Multimodal Biometrics: Combining multiple biometric modalities (e.g., fingerprint and facial recognition) will enhance accuracy and security, making it even more difficult for fraudsters to bypass authentication systems.
- Behavioral Biometrics: This technology analyzes patterns in user behavior, such as typing speed and mouse movements, to provide continuous authentication. This can add an extra layer of security to AML processes.
- Integration with AI: AI-driven biometric systems will improve the accuracy and speed of identity verification, reducing false positives and enhancing the overall efficiency of AML efforts.
The Intersection of Cybersecurity and AML
Cybersecurity and AML are closely intertwined, as both aim to protect financial systems from illicit activities. Effective cybersecurity measures are essential for safeguarding sensitive AML data and ensuring the integrity of AML processes.
Several trends are shaping the future of cybersecurity in the context of AML:
- Zero Trust Architecture: This security model assumes that threats could be both external and internal, requiring continuous verification of user identities and access privileges. This approach will be crucial for protecting AML data.
- AI and Machine Learning: These technologies will play a significant role in identifying and mitigating cyber threats in real-time, enhancing the security of AML systems.
- Quantum Cryptography: As quantum computing advances, quantum cryptography will provide unprecedented levels of security for AML data, making it virtually impossible for cybercriminals to decrypt sensitive information.
Cross-Border Data Sharing and AML
Money laundering is a global issue that often involves cross-border transactions. Effective AML efforts require international cooperation and data sharing to track and combat illicit activities across different jurisdictions.
Blockchain technology provides a secure and transparent way to share data across borders, ensuring the integrity and traceability of financial transactions. Secure data exchange platforms enable financial institutions to share AML data securely, facilitating better collaboration and information sharing.
Several trends are expected to shape the future of cross-border data sharing in AML. Blockchain technology will provide a secure and transparent way to share data across borders, ensuring the integrity and traceability of financial transactions. The development of global data standards will enable more seamless and efficient data sharing between financial institutions and regulatory bodies, enhancing the effectiveness of AML efforts. Additionally, privacy-enhancing technologies (PETs), such as homomorphic encryption and secure multi-party computation, will allow financial institutions to share data securely while preserving privacy, addressing concerns about data protection and compliance with privacy regulations.
Leveraging Advanced AML Solutions for Future Compliance
The convergence of advanced technologies and evolving regulations is set to redefine the landscape of AML. AI, machine learning, blockchain, and biometric authentication will play crucial roles in enhancing the efficiency and accuracy of AML processes. However, a holistic approach that combines these technologies with stringent regulations and human expertise is essential. Predictions for the next decade include increased use of AI, real-time monitoring, global standardization of k, integration of privacy-enhancing technologies, and the rise of Regtech solutions. The AI in the FinTech market is expected to grow from $7.3 billion in 2020 to $26.7 billion by 2026, highlighting the increasing adoption of AI technologies in the financial sector.
Sanction Scanner is at the forefront of this technological revolution, offering advanced AML solutions that integrate AI, machine learning, and real-time monitoring to help financial institutions stay compliant with evolving regulations. By leveraging Sanction Scanner's innovative tools, institutions can enhance their AML efforts, reduce compliance costs, and stay ahead of emerging threats. To see how Sanction Scanner can transform your AML strategy, request a demo today and experience the future of AML compliance firsthand.
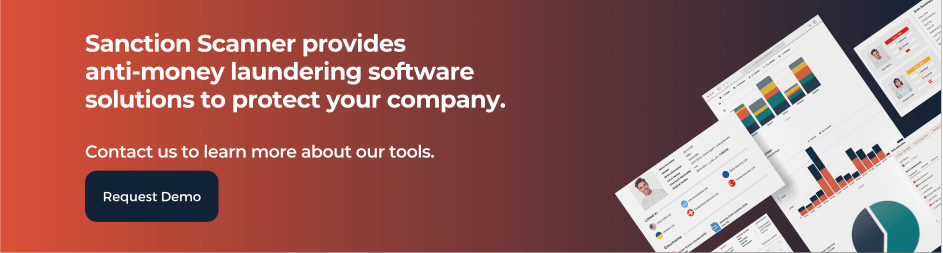