To prevent money laundering, it's crucial for all financial institutions to have a risk-based AML program in place that complies with regulations and addresses laundering and terrorist financing risks. A comprehensive AML program includes Customer Risk Assessment, Customer Due Diligence, Enhanced Due Diligence, transaction monitoring, adverse media screenings, and other monitoring and screening operations.
Transaction monitoring systems are designed to generate warning alarms if any suspicious activity is detected. These alarms help companies identify potential money laundering or terrorist financing activities and take the necessary steps to mitigate the risks. However, it's important to note that not every alarm may indicate money laundering. Transaction monitoring tends to flag any unusual activity, which can result in false alarms being generated, even in situations that pose no actual risk.
What is Transaction Monitoring?
Transaction monitoring is a key part of AML compliance. Financial institutions use transaction monitoring systems to continuously analyze financial transactions for potentially suspicious activity related to money laundering, terrorist financing, or other financial crimes. The system generates alerts for transactions that meet certain criteria, which are then investigated by AML analysts. Transaction monitoring is critical for regulatory compliance and detecting and preventing financial crimes. It requires ongoing monitoring and effective systems, and trained personnel.
What are False Positive Alarms in Transaction Monitoring?
In the realm of transaction monitoring, false positive alarms can prove to be a challenging issue. These types of alarms are triggered when the AML software generates an alert or alarm based on a transaction that appears to be suspicious, but in reality, is a legitimate transaction. Such false alarms can prove to be costly for financial institutions, as they can result in the unnecessary allocation of resources to investigate the transaction, which can cause delays in legitimate transactions.
The issue of false positives is not unique to transaction monitoring and is prevalent in many detection systems. In transaction monitoring, false positives can occur due to several reasons. One of the reasons is when a transaction has a common pattern that appears in legitimate transactions, such as regular payments to a vendor or regular salary payments. The system may flag such transactions as suspicious, even though they are legitimate.
Another reason for false positives is when the system lacks proper data integration. Data integration is crucial in transaction monitoring as it enables the system to correlate transactions across various accounts and transactions. If the data integration is not done correctly, it can lead to inaccurate alerts. Moreover, false positives can occur when the system has outdated or inadequate rules. Financial institutions must update their rules regularly to reflect changes in the regulatory environment and to adapt to new emerging risks.
The impact of false positives can be significant, resulting in increased costs and reduced efficiency. To mitigate the risk of false positives, financial institutions can adopt several strategies. One of the strategies is to review and analyze historical data to identify patterns and adjust the system's rules accordingly. Financial institutions can also invest in advanced technologies such as artificial intelligence and machine learning to improve the accuracy of the system.
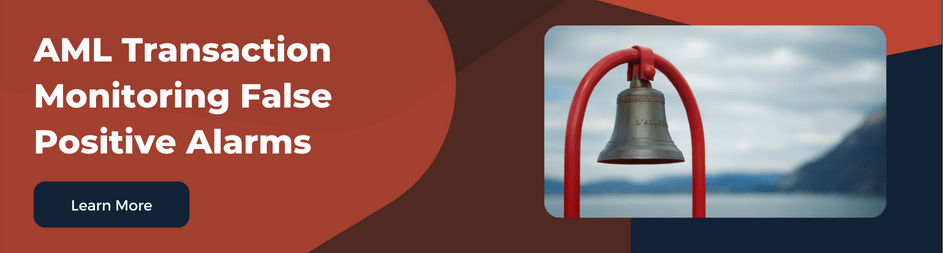
Causes of False Positive Alarms in AML Transaction Monitoring
Several factors can contribute to false positive alarms in AML transaction monitoring. Here are some of the main causes:
- Incomplete or outdated data: AML transaction monitoring systems rely on accurate and up-to-date data to identify suspicious activity. If the system has incomplete or outdated data, it may generate false positive alarms.
- Unusual but legitimate transactions: Some legitimate transactions may appear unusual to the AML transaction monitoring system. For example, a customer may make a large cash deposit as part of a legitimate business transaction. However, if the AML system is not configured to recognize this type of activity, it may generate a false positive alarm.
- Lack of context: AML transaction monitoring systems rely on context to identify suspicious activity. If the system does not have enough context around a transaction, it may generate a false positive alarm. For example, if a customer suddenly makes a large transfer to a foreign account, the system may flag it as suspicious. However, if the customer has a legitimate reason for the transfer, such as paying for a vacation, the system may not have the context to recognize this.
- System configuration: The way an AML transaction monitoring system is configured can also contribute to false positive alarms. If the system is configured to be overly sensitive, it may generate more false positive alarms. On the other hand, if it is not sensitive enough, it may miss suspicious activity.
Businesses are required by regulatory guidelines to produce a Suspicious Activity Report (SAR) for any transactions that appear suspicious. However, this process can sometimes be problematic, especially when innocent transactions are flagged as false positives, triggering unnecessary alarms. This can result in delays in reporting actual criminal activities and businesses wasting their time. False positives can also lead to innocent customers being treated as guilty, resulting in a negative customer experience. Unfortunately, many businesses end up losing valuable customers due to false positives. Therefore, it is crucial for businesses to carefully review and analyze transactions before flagging them as suspicious to minimize the risk of false positives.
Reducing False Positives
- Fine-tune risk assessment models: Implement sophisticated risk assessment models that take into account various factors such as transaction history, customer behavior, and geographical patterns. By customizing risk profiles for different customer segments, businesses can more accurately identify suspicious activities and minimize false positives.
- Implement machine learning and AI technologies: Leverage advanced technologies like machine learning and artificial intelligence to enhance the accuracy of AML monitoring. These technologies can analyze large volumes of data, identify patterns, and make real-time risk assessments, resulting in more precise detection of suspicious transactions and a reduction in false positives.
- Collaborate with industry peers and authorities: Establish partnerships and information-sharing initiatives with other financial institutions, regulatory bodies, and law enforcement agencies. Sharing insights, best practices, and intelligence can help identify emerging trends and potential threats, enabling more effective AML strategies and reducing false positives.
- Continuous staff training and awareness: Provide regular training to employees involved in AML compliance to ensure they stay updated on the latest regulations, typologies of financial crimes, and emerging risks. Well-informed staff can make more accurate assessments and reduce false positives through a better understanding of suspicious indicators and scenarios.
- Enhance data quality and accuracy: Ensure the integrity and quality of data used for AML monitoring. Implement robust data management processes, including data cleansing, validation, and regular updates, to minimize errors and inconsistencies. High-quality data enables more accurate analysis and reduces false positive alerts.
- Implement scenario-based monitoring: Develop and implement scenario-based monitoring systems that focus on specific types of suspicious activities relevant to your business and customer base. By tailoring monitoring scenarios to your specific risk profile, you can better identify genuine risks while minimizing false positives associated with generic rules.
- Regularly review and update AML policies and procedures: Conduct periodic reviews of your AML policies and procedures to ensure they align with the evolving regulatory landscape. Stay informed about industry best practices and regulatory guidelines, and make necessary updates to your systems and processes accordingly. This helps to improve the accuracy of AML monitoring and reduce false positives.
How does Sanction Scanner Help to Reduce False Positives?
Sanction Scanner is a comprehensive compliance solution that helps businesses meet their regulatory obligations by providing real-time screening of transactions against global sanctions and watchlists. This powerful software allows businesses to create custom rules and scenarios to identify potential matches and minimize false positives, while also providing additional search options like date of birth, ID number, or passport number to reduce the likelihood of false alarms. By using Sanction Scanner, businesses can improve their transaction monitoring and stay up-to-date with global compliance regulations.