In the intricate world of Anti-Money Laundering (AML) compliance, financial institutions are constantly striving to strike a balance between being vigilant and being efficient. One of the most persistent challenges they face is the issue of AML false positives – a problem that not only affects operational efficiency but also has broader implications for customer relationships and regulatory compliance.
What are AML False Positives?
AML false positives occur when a legitimate transaction or customer behavior is incorrectly flagged as suspicious by an AML monitoring system. These systems are designed to detect patterns indicative of money laundering or other financial crimes, but they are not infallible. When a false positive happens, it means that the system has identified an activity that, upon further investigation, turns out to be innocuous and not related to any illicit activity.
The term "false positive" is borrowed from the field of statistics and diagnostic testing, where it refers to a test result that wrongly indicates the presence of a condition when it is not actually present. In the context of AML, a false positive can be thought of as a "false alarm" – an alert that triggers a review process, which can be time-consuming and resource-intensive, only to conclude that there was no real threat or violation.
False positives are a significant concern for financial institutions because they can lead to wasted resources, unnecessary customer friction, and a diversion of attention from genuine threats. The goal of any AML program is to accurately identify and report suspicious activities while minimizing the number of false positives. Achieving this balance is critical for maintaining an effective and efficient AML compliance program.
Why Do AML False Positives Happen?
There are several factors that contribute to the high rate of false positives in AML processes:
- Overly Sensitive Detection Systems: AML systems are often configured to be highly sensitive to a wide range of risk indicators to ensure that no potential threat goes unnoticed. This heightened sensitivity, while well-intentioned, can lead to a large number of transactions being flagged that are, in fact, legitimate.
- Lack of Tailored Rules and Scenarios: Financial institutions may use generic rules and scenarios that do not account for the specific risk profile of their customers or the unique patterns of legitimate transactions within their business. Without customization, these rules can trigger alerts for normal customer behavior.
- Inadequate Data Quality: The quality of data fed into AML systems significantly impacts their performance. Inaccurate, incomplete, or outdated information can lead to misidentification of transactions as suspicious. This includes errors in customer databases, transaction records, or third-party data sources.
- Rapidly Evolving Financial Crimes: Criminals are constantly devising new methods to launder money, which means AML systems must continuously adapt. However, there can be a lag in updating systems to reflect emerging typologies, during which time they may generate false positives for unfamiliar but legitimate transaction patterns.
- Complexity of Financial Ecosystems: The increasing complexity of financial products, services, and channels through which transactions occur adds to the challenge. Cross-border transactions, multiple currencies, and the rise of fintech solutions can all complicate the detection process.
- Human Factor: Finally, the human element cannot be overlooked. Analysts reviewing alerts may have varying levels of expertise and judgment, which can influence the rate of false positives. Additionally, manual processes are prone to error, contributing further to the issue.
How to Reduce False Positives in 5 Steps
Reducing the number of false positives in AML processes is not only beneficial for improving operational efficiency but also for better compliance and customer experience. Here are five strategic steps that financial institutions can take to minimize the occurrence of false positives:
1. Enhance Data Quality and Integration
The foundation of any effective AML system is high-quality data. Financial institutions should invest in improving the accuracy, completeness, and timeliness of the data they collect. This includes regular updates to customer information and integrating data from various sources to create a more comprehensive view of customer activity. Enhanced data quality allows for more precise risk assessments and reduces the likelihood of false alerts.
2. Implement Advanced Analytical Techniques
Utilizing machine learning and artificial intelligence can significantly improve the detection of genuine suspicious activities while reducing false positives. These technologies can analyze large volumes of transactions in real-time, learn from historical data, and adapt to new patterns of money laundering. By recognizing the nuances in transaction behaviors, they can discern between legitimate and suspicious activities more effectively than traditional rule-based systems.
3. Customize Detection Scenarios
Financial institutions should tailor their AML systems to reflect their specific risk profile, customer base, and the nature of their transactions. Customizing detection scenarios and thresholds to align with the institution's unique environment can reduce irrelevant alerts. This involves conducting regular risk assessments and updating scenarios to keep pace with changes in customer behavior and emerging threats.
4. Streamline Alert Investigation Processes
Improving the efficiency of the alert investigation process can help compliance teams focus on genuine risks. This can be achieved by implementing a risk-based approach to prioritize alerts, automating routine tasks, and providing ongoing training to analysts to enhance their decision-making skills. Streamlining these processes helps to reduce the backlog of alerts and ensures that true positives are identified and acted upon promptly.
5. Regularly Review and Optimize AML Systems
AML systems and processes should not be static. Regular reviews and optimization are crucial to ensure they remain effective over time. This includes back-testing the system against past false positives to identify patterns that can be adjusted, conducting periodic model validations, and staying abreast of regulatory changes and criminal typologies. Continuous improvement of the AML framework is key to maintaining a balance between vigilance and operational efficiency.
Click here to learn how AML transaction monitoring reduces false positives.
Reduce False Positives with Sanction Scanner
In the quest to combat money laundering effectively, the role of advanced technology solutions cannot be overstated. Sanction Scanner emerges as a powerful ally in this fight, offering cutting-edge tools designed to minimize the headache of AML false positives. With its sophisticated algorithms and user-friendly interface, Sanction Scanner provides a seamless integration into your existing AML framework, enhancing accuracy and efficiency.
Sanction Scanner's platform is engineered to address the common pain points that lead to false positives. By leveraging comprehensive databases and real-time analysis, it ensures that your financial institution is equipped with up-to-date information, reducing the chances of outdated or incomplete data compromising your AML efforts. The system's advanced analytics are tailored to adapt to the unique patterns of your transactions, providing a customized approach that aligns with your specific risk profile.
Moreover, the intuitive design of Sanction Scanner's tools empowers your compliance team to conduct thorough investigations with greater speed and precision. The platform's automation capabilities free up valuable resources, allowing your analysts to concentrate on the alerts that truly matter.
Don't let false positives undermine your compliance efforts. Ready to see the impact on your AML strategy? Request a demo today and explore how Sanction Scanner can help you maintain compliance and focus on real financial crime risks.
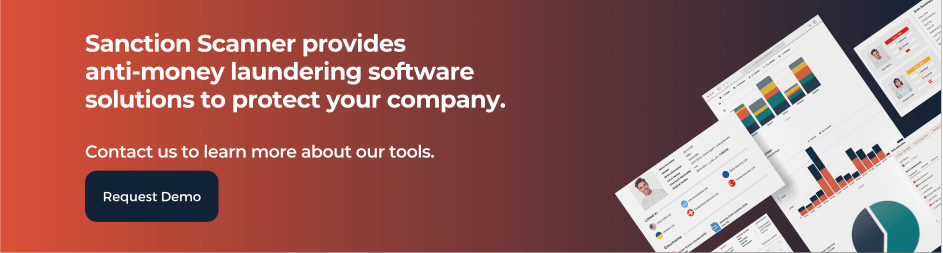